Fusing Local Similarities for Retrieval-based 3D Orientation Estimation of Unseen Objects
ECCV 2022
Abstract
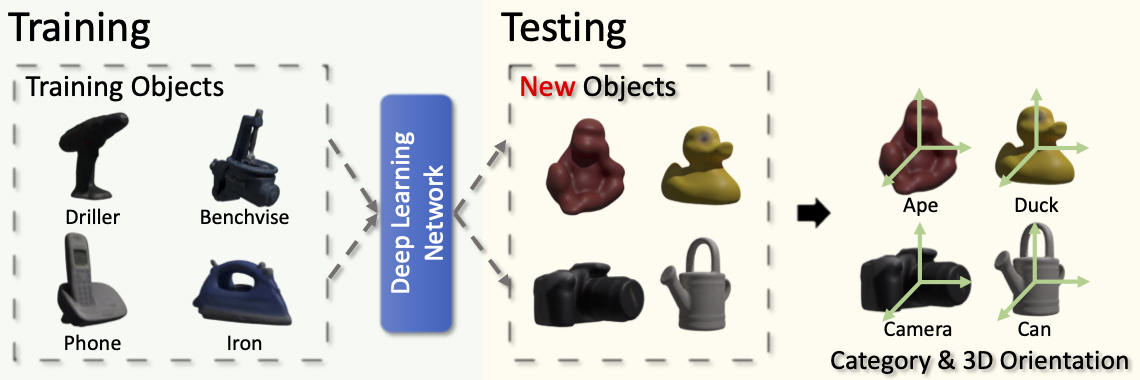
Background:
Estimating the 3D orientation of objects from an image is pivotal to many computer vision and robotics tasks. Most learning-based methods assume that the training data and testing data contain exactly the same objects or similar objects from the same category. However, this assumption is often violated in real-world applications, such as robotic manipulation, where one would typically like the robotic arm to be able to handle previously-unseen objects without having to re-train the network for them.
Our Contributions:
- We estimate the 3D orientation of previously-unseen objects by introducing an image retrieval framework based on multi-scale local similarities.
- We develop a similarity fusion module, robustly predicting an image similarity score from multi-scale pairwise feature maps.
- We design a fast retrieval strategy that achieves a good trade-off between the 3D orientation estimation accuracy and efficiency.
Method Overview
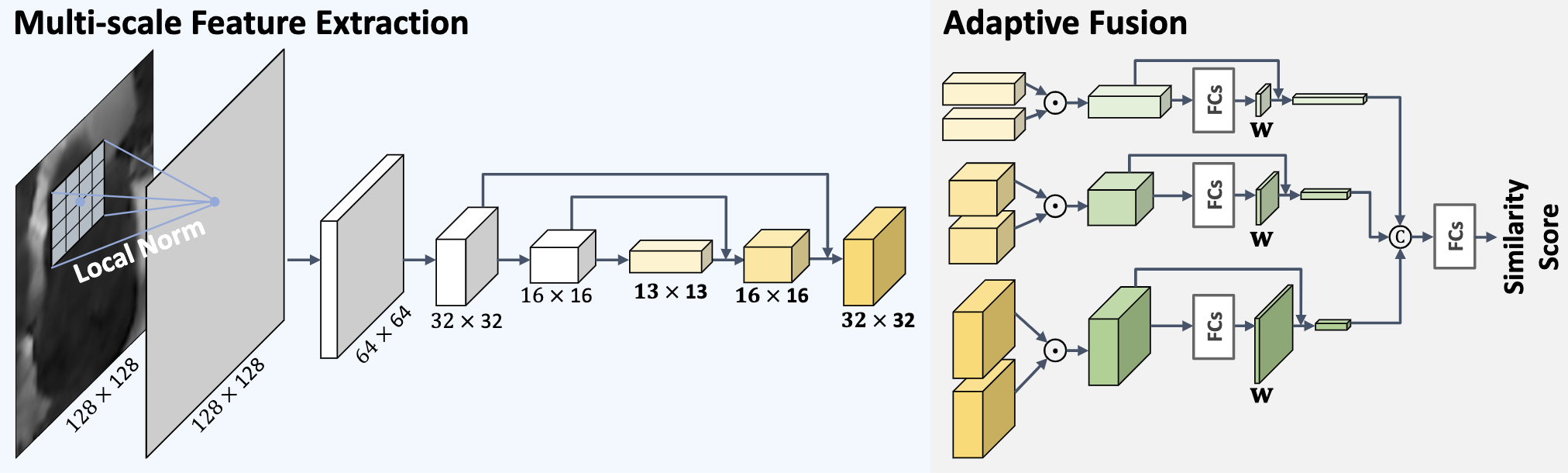
Results on LineMOD and LineMOD-O
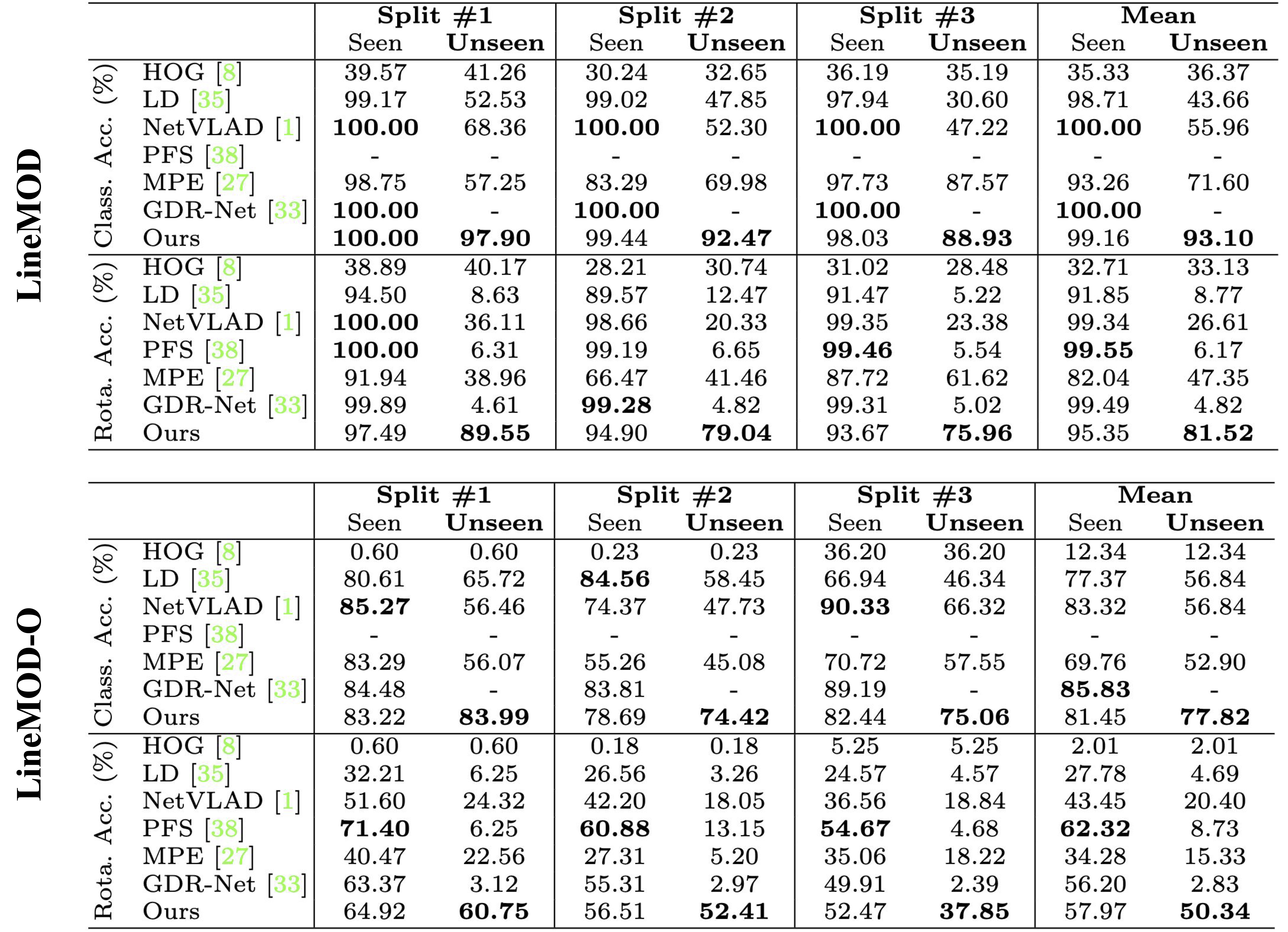
Visualization
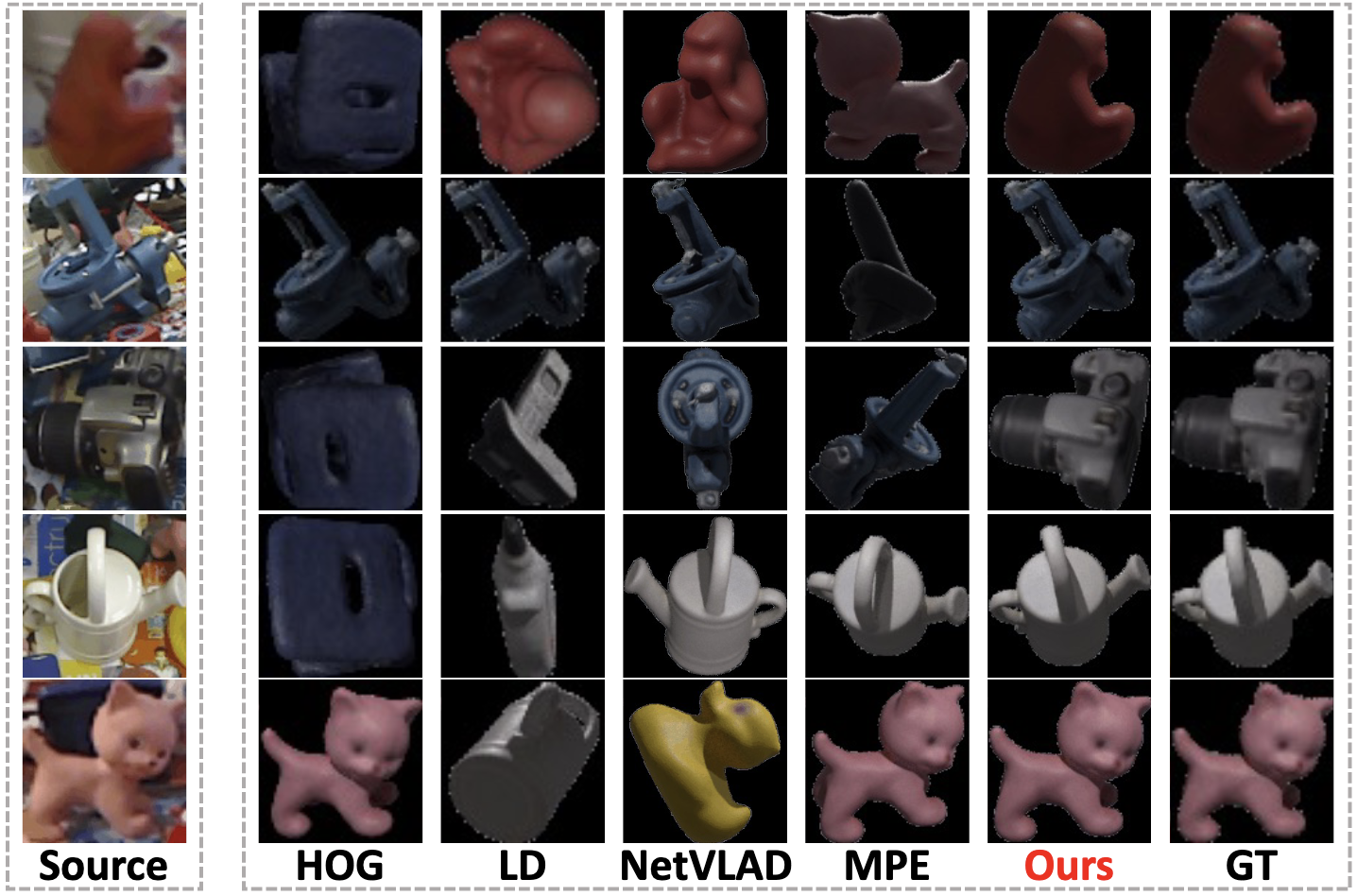
Citation
@article{zhao2022fusing, title={Fusing Local Similarities for Retrieval-based 3D Orientation Estimation of Unseen Objects}, author={Zhao, Chen and Hu, Yinlin and Salzmann, Mathieu}, journal={arXiv preprint arXiv:2203.08472}, year={2022} } }
Contact
If you have any question, please contact Chen ZHAO at chen.zhao@epfl.ch.