Progressive Correspondence Pruning by Consensus Learning
1. Computer Vision Laboratory, École polytechnique fédérale de Lausanne (EPFL)
2. SenseTime Research
3. The Chinese University of Hong Kong
4. Qing Yuan Research Institute, Shanghai Jiao Tong University
2. SenseTime Research
3. The Chinese University of Hong Kong
4. Qing Yuan Research Institute, Shanghai Jiao Tong University
Abstract [Full Paper]
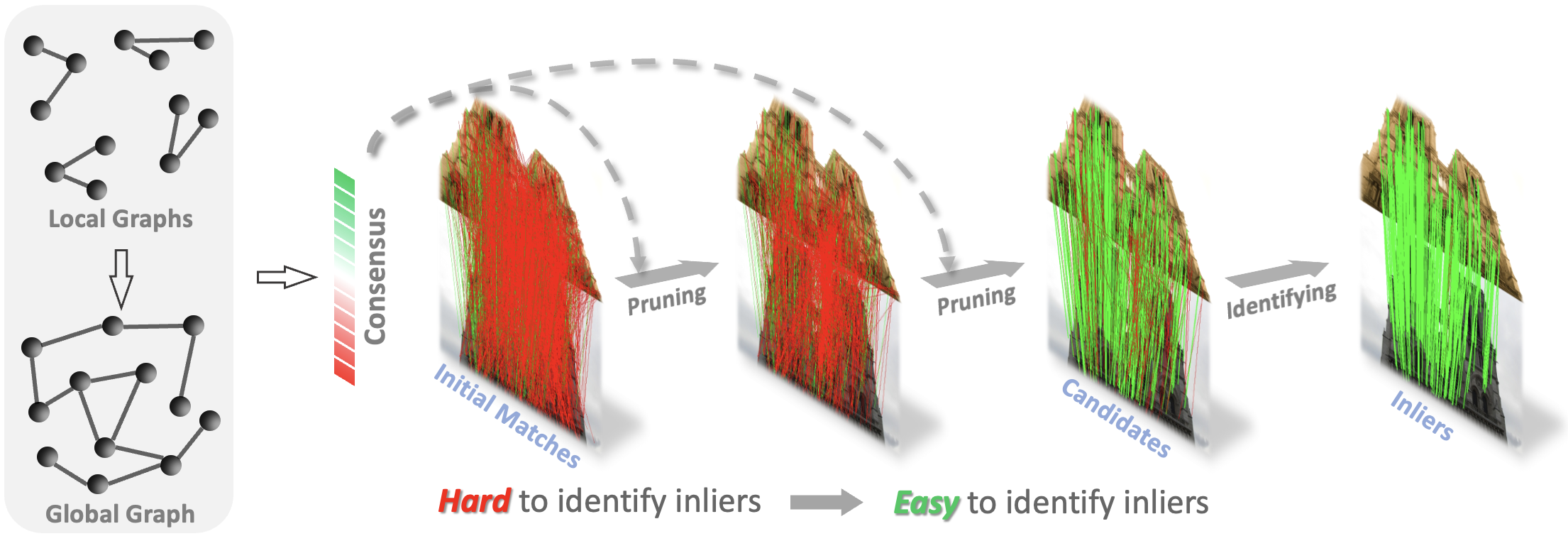
Background:
Correspondence selection aims to correctly select the consistent matches (inliers) from an initial set of putative correspondences. The selection is challenging since putative matches are typically extremely unbalanced, largely dominated by outliers, and the random distribution of such outliers further complicates the learning process for learning-based methods.
Our Contributions:
- We propose to progressively prune correspondences for better inlier identification, which alleviates the effects of unbalanced initial matches and random outlier distribution.
- We introduce a local-to-global consensus learning network for robust correspondence pruning, achieved by establishing dynamic graphs on-the-fly and estimating both local and global consensus scores to prune correspondences.
Method Overview
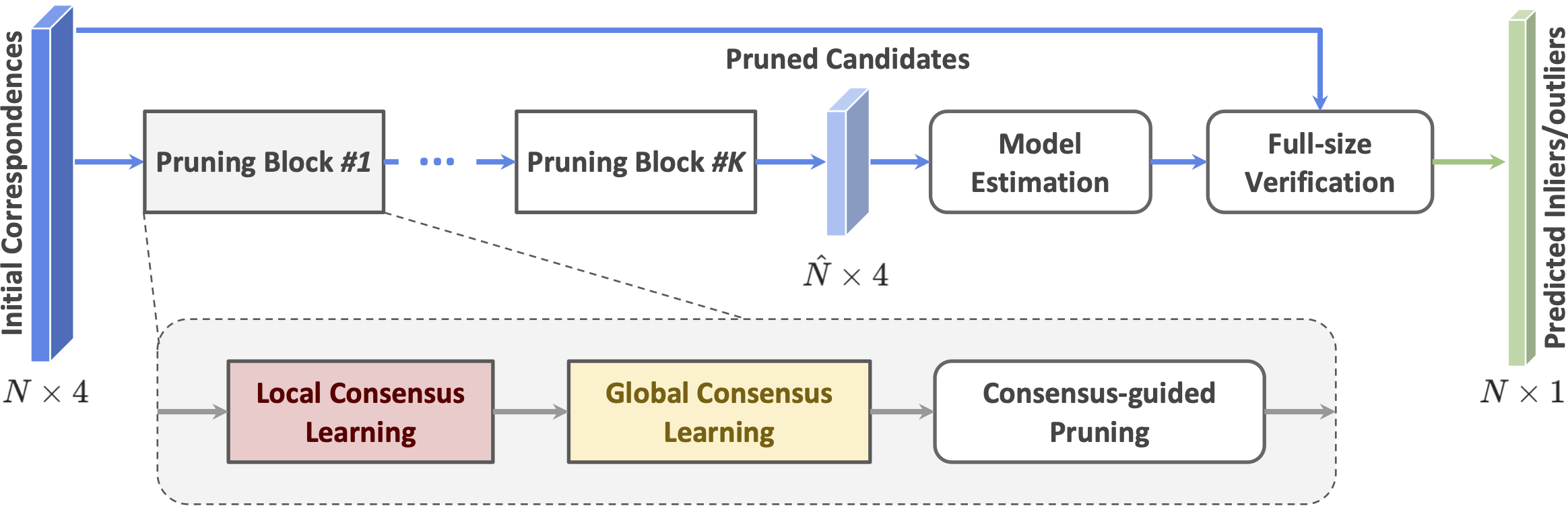
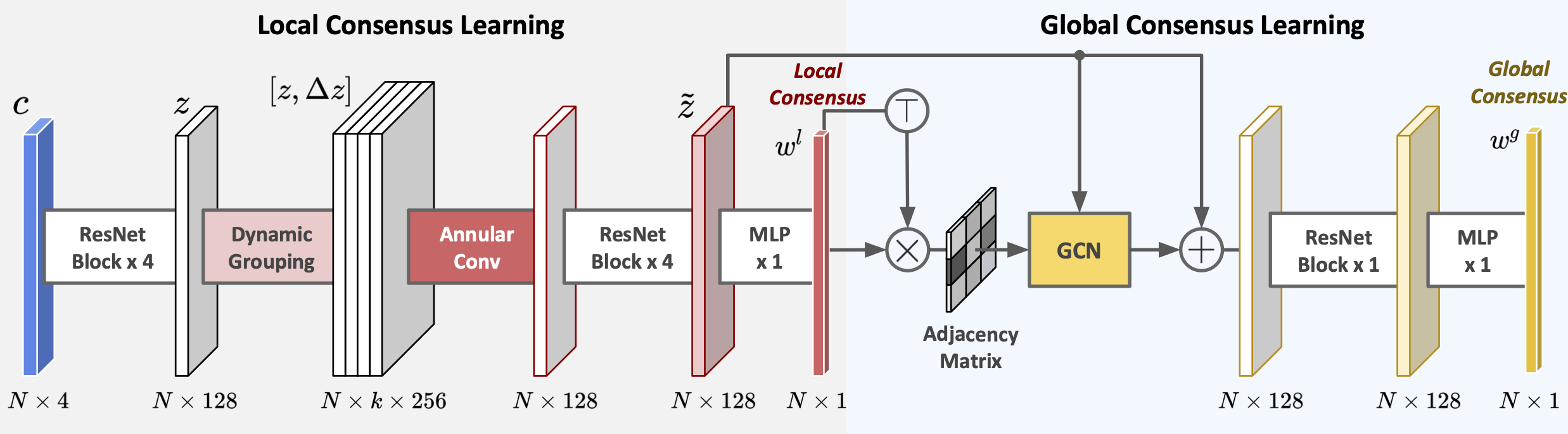
Results on YFCC100M and SUN3D
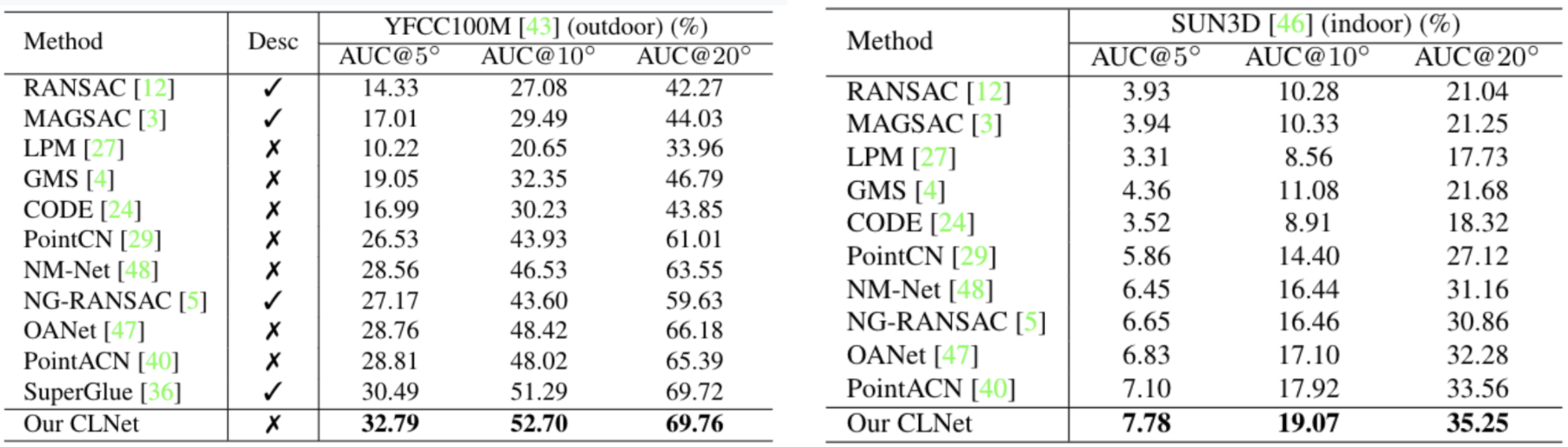
Visualization
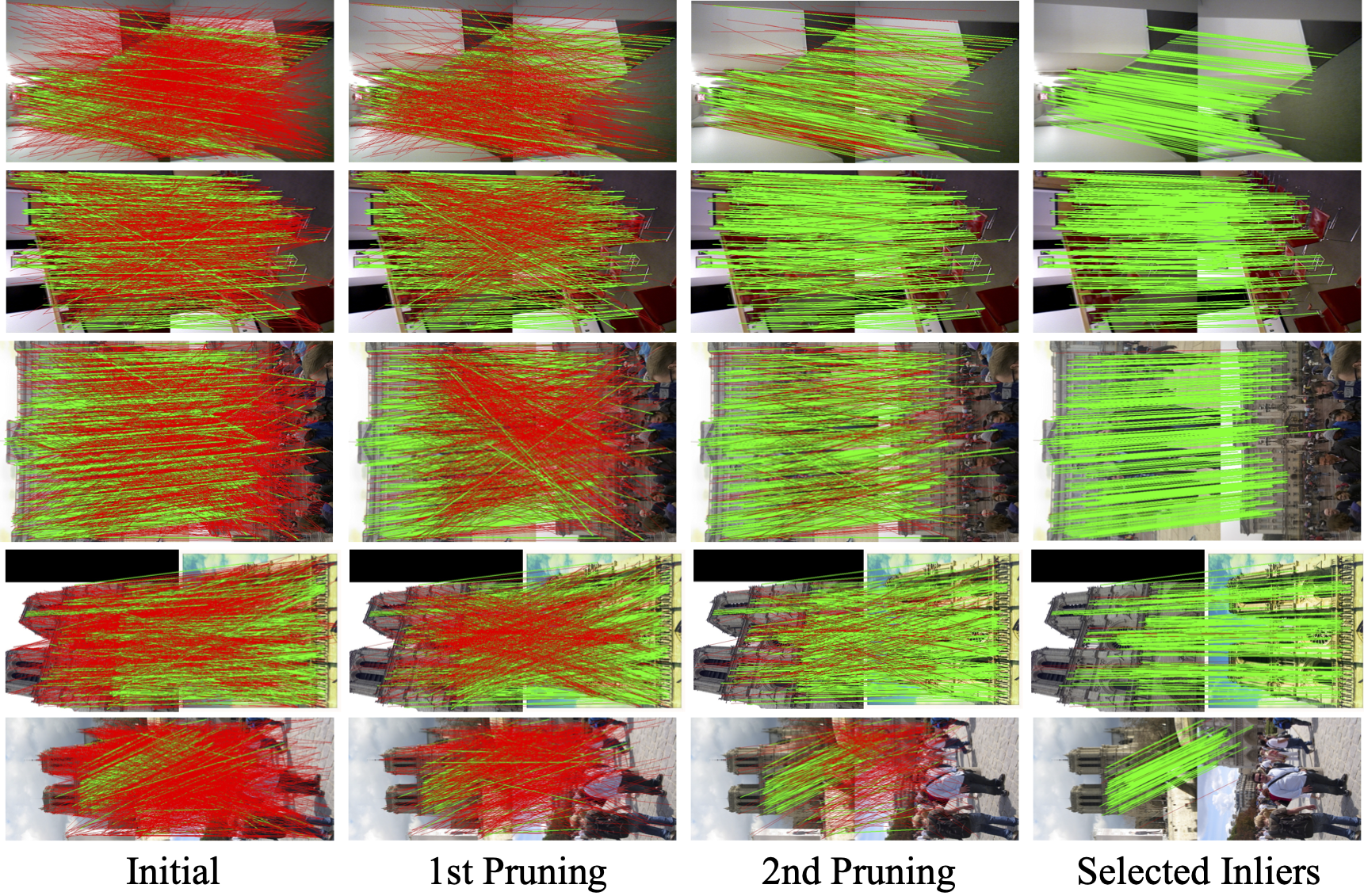
Links
Citation
@article{zhao2021consensus, title={Progressive Correspondence Pruning by Consensus Learning}, author={Zhao, Chen and Ge, Yixiao and Zhu, Feng and Zhao, Rui and Li, Hongsheng and Salzmann, Mathieu}, journal={arXiv preprint arXiv:2101.00591}, year={2021} } }
Contact
If you have any question, please contact Chen ZHAO at chen.zhao@epfl.ch.