3D-Aware Hypothesis & Verification for Generalizable Relative Object Pose Estimation
ICLR 2024
Abstract [Full Paper]
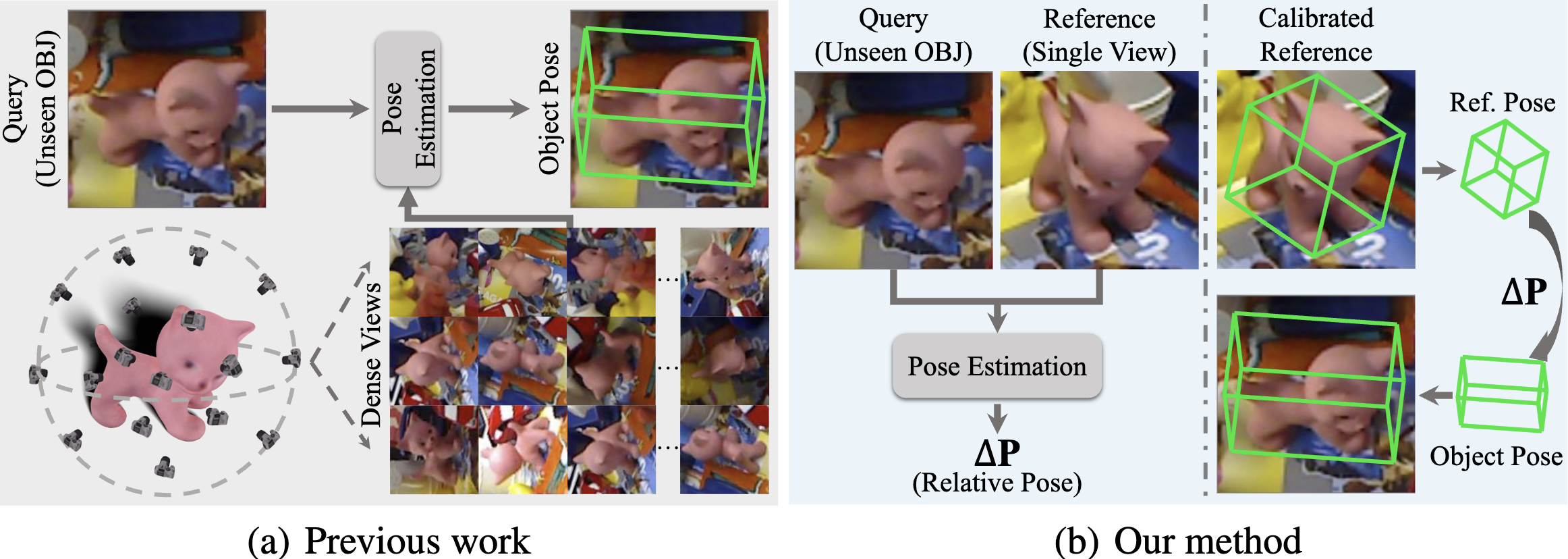
Prior methods that tackle the problem of generalizable object pose estimation highly rely on having dense views of the unseen object. By contrast, we address the scenario where only a single reference view of the object is available. Our goal then is to estimate the relative object pose between this reference view and a query image that depicts the object in a different pose. In this scenario, robust generalization is imperative due to the presence of unseen objects during testing and the large-scale object pose variation between the reference and the query. To this end, we present a new hypothesis-and-verification framework, in which we generate and evaluate multiple pose hypotheses, ultimately selecting the most reliable one as the relative object pose. To measure reliability, we introduce a 3D-aware verification that explicitly applies 3D transformations to the 3D object representations learned from the two input images. Our comprehensive experiments on the Objaverse, LINEMOD, and CO3D datasets evidence the superior accuracy of our approach in relative pose estimation and its robustness in large-scale pose variations, when dealing with unseen objects.
Method Overview
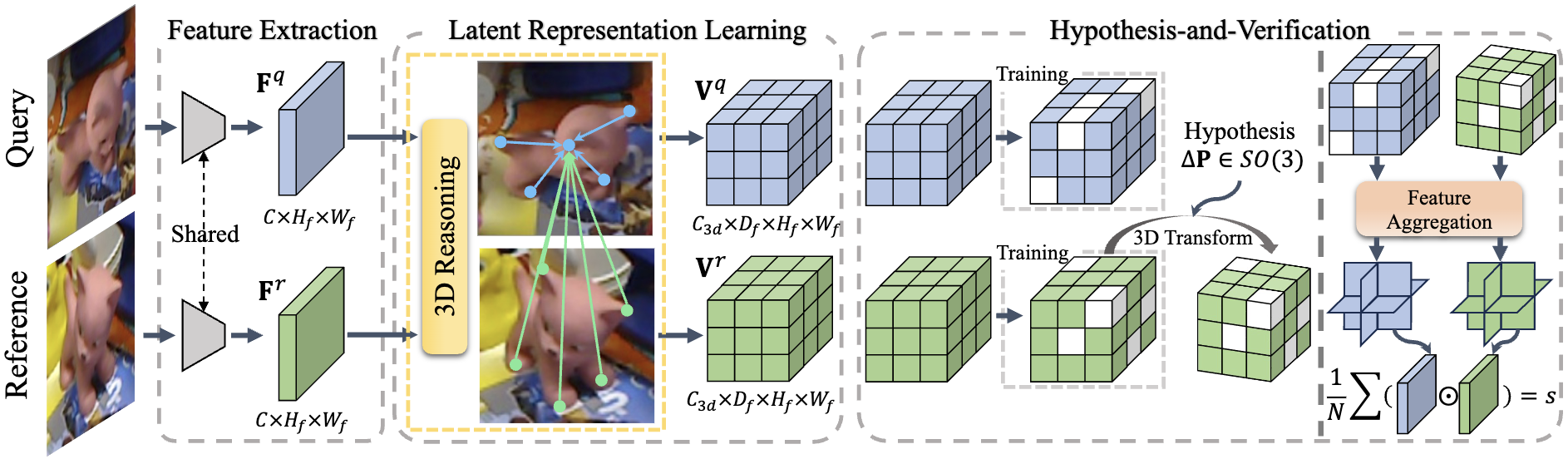
A hypothesis $\Delta{\mathbf{P}}$ is randomly sampled and its accuracy is measured as a score $s$. To explicitly integrate 3D information, we perform the verification over a 3D object representation indicated as a learnable 3D volume. The sampled hypothesis is coupled with the learned representation via a 3D transformation over the reference 3D volume. We learn the 3D volumes from the 2D feature maps extracted from the RGB images by introducing a 3D reasoning module. To improve robustness, we randomly mask out some blocks colored in white during training.
Results on Objaverse and LINEMOD
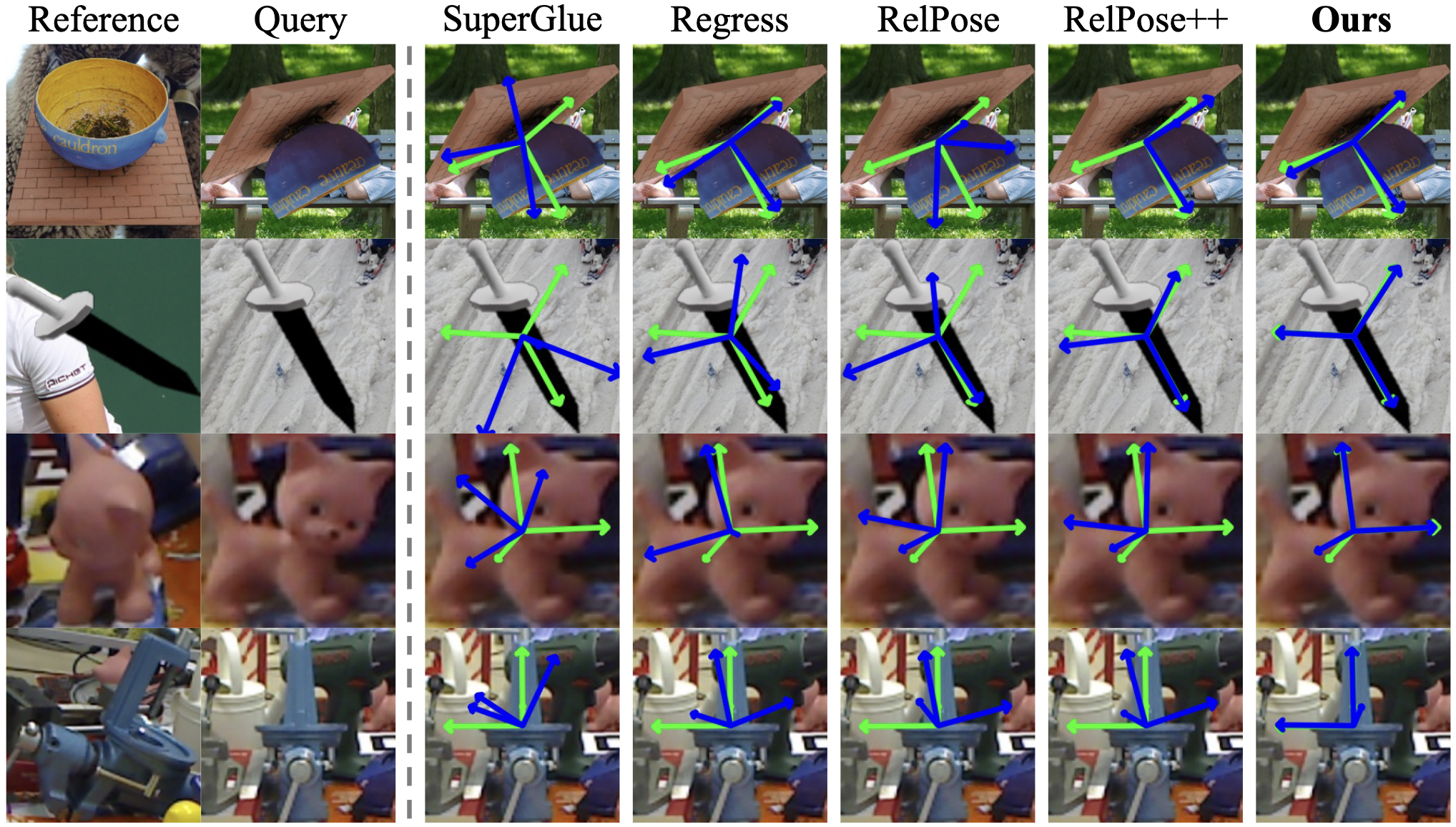

Citation
@article{zhao20233d, title={3D-Aware Hypothesis \& Verification for Generalizable Relative Object Pose Estimation}, author={Zhao, Chen and Zhang, Tong and Salzmann, Mathieu}, journal={Proceedings of the International Conference on Learning Representations}, year={2024} }
Contact
If you have any question, please contact Chen ZHAO at chen.zhao@epfl.ch.